Understanding the power of machine learning can mean the difference between soaring ahead of the competition or being left in the shadows of obscurity. Welcome to a world where harnessing the potential of data-driven insights and intelligent algorithms holds the promise of transforming your e-commerce endeavors into a resounding success.
Brace yourself as we unravel the vital importance of Machine Learning in E-commerce, revealing how it can truly make or break your journey in the fierce landscape of digital retail. Get ready to discover the strategic weapon that will propel you to the forefront of the competition.
Are you prepared to crack the code? Let’s embark on this transformative journey together!
Introduction to Machine Learning in E-Commerce
In today’s rapidly evolving digital landscape, technology continues to reshape the way businesses operate, and machine learning stands at the forefront of this transformative journey. In the context of e-commerce, machine learning opens up a world of possibilities, offering invaluable opportunities for businesses to optimize their operations, elevate customer experiences, and gain a competitive edge.
What is Machine Learning and What Can It Do For E-Commerce?
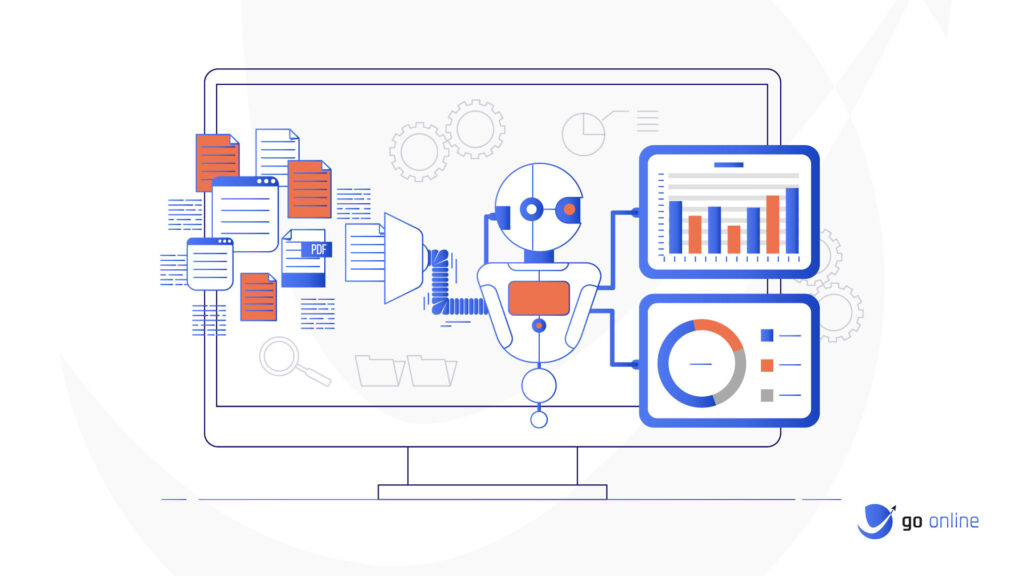
At its core, machine learning is a subset of artificial intelligence that enables computer systems to learn from data patterns and experiences, without the need for explicit programming.
It’s like having an intelligent assistant that can identify hidden insights from vast datasets, predict customer behavior, and make informed decisions based on the information it learns.
Now, you might wonder, “What does this mean for the e-commerce industry?” Well, it means a myriad of exciting prospects that can revolutionize the way e-commerce businesses function and engage with their customers.
5 benefits Of Using Machine Learning In E-Commerce
In the ever-changing digital landscape, machine learning takes the lead as a transformative technology reshaping industries, including e-commerce. Embracing the power of machine learning, online retailers can tap into a world of possibilities, streamlining operations and providing exceptional customer experiences.
From tailored product recommendations and boosted conversion rates to precise and accurate customer segmentation,, reliable demand forecasting, and fortified fraud detection and customer service automation, machine learning unlocks a myriad of advantages, propelling e-commerce businesses to thrive in a fiercely competitive market.
Enchanced Personalization
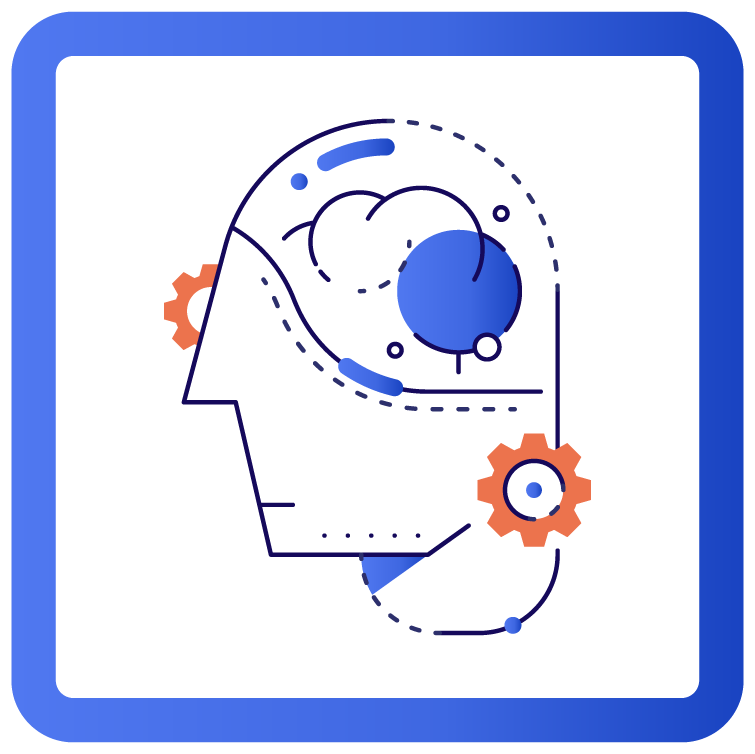
Imagine stepping into a brick-and-mortar store, and the salesperson knows your preferences, likes, and dislikes, providing tailored recommendations just for you. Machine learning brings this level of personalized experience to the digital realm.
By analyzing customer data, such as browsing history, purchase behavior, and demographics, e-commerce platforms can offer highly personalized product recommendations, content, and promotions.
This enhances customer engagement and loyalty, leading to increased sales and repeat business.
Increased Conversion
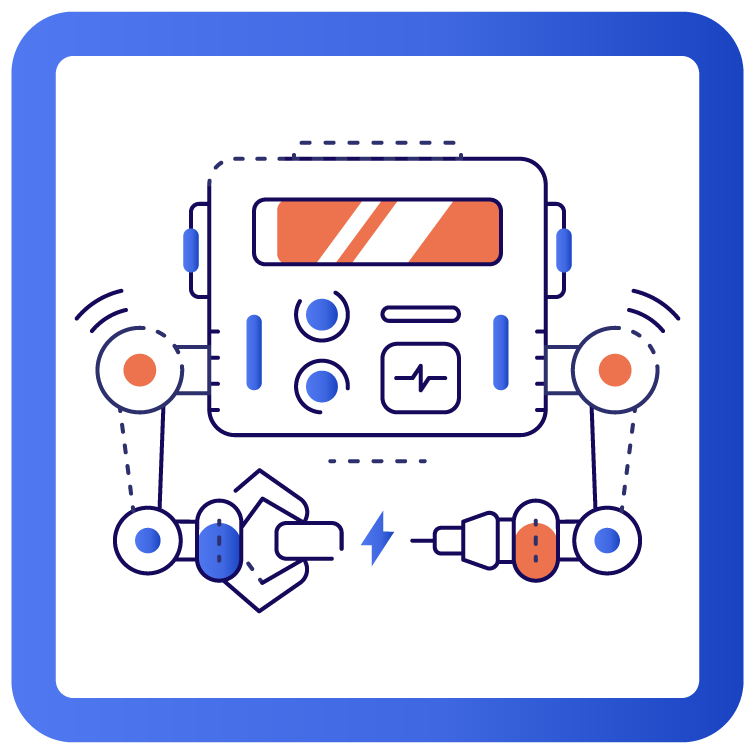
Turning website visitors into customers is a key challenge for e-commerce businesses. Machine learning comes to the rescue by identifying patterns that influence purchase decisions.
By understanding customer behavior and preferences, e-commerce platforms can optimize their website design, product positioning, and marketing strategies, leading to higher conversion rates and improved sales performance.
Efficient Customer Segmentation
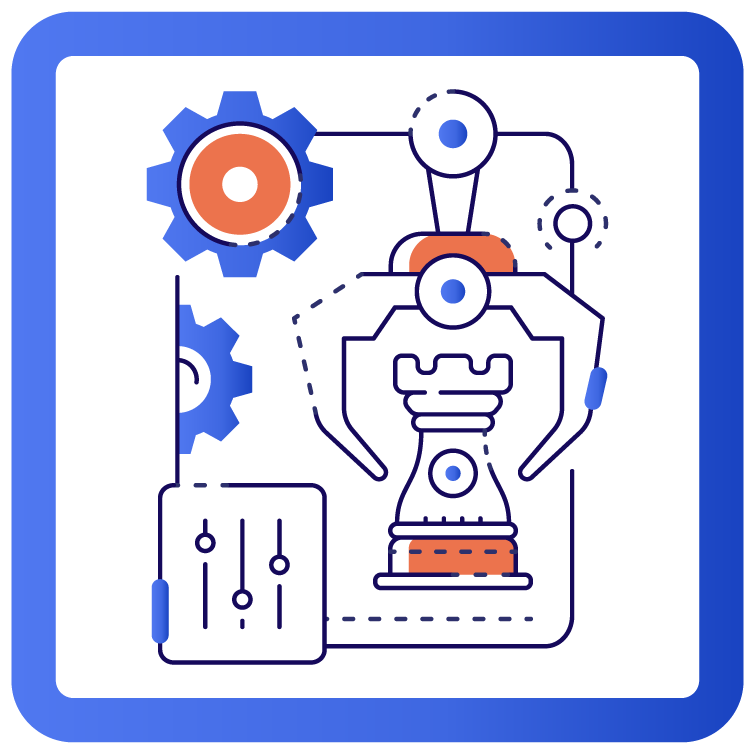
Gone are the days of one-size-fits-all marketing strategies. Machine learning empowers businesses to do more accurate customer segmentation of their customer base more effectively and in real-time. By categorizing customers based on their behaviors and preferences, businesses can target them with personalized marketing campaigns, tailored offers, and relevant content.
This level of segmentation enhances customer engagement and retention, ultimately driving business growth.
Demand Forecasting
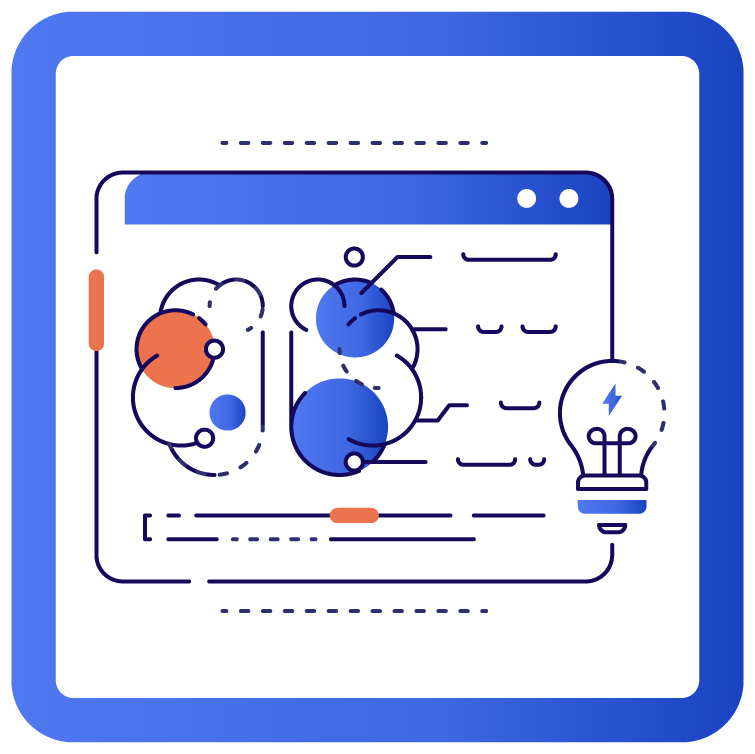
Accurate demand forecasting is a critical aspect of e-commerce operations. Machine learning models analyze historical sales data, seasonal trends, and external factors to predict future demand more accurately.
This foresight enables online businesses to to manage inventory efficiently, optimize pricing strategies, and ensure they meet customer demands effectively.
Fraud DetectionCustomer Service Automation
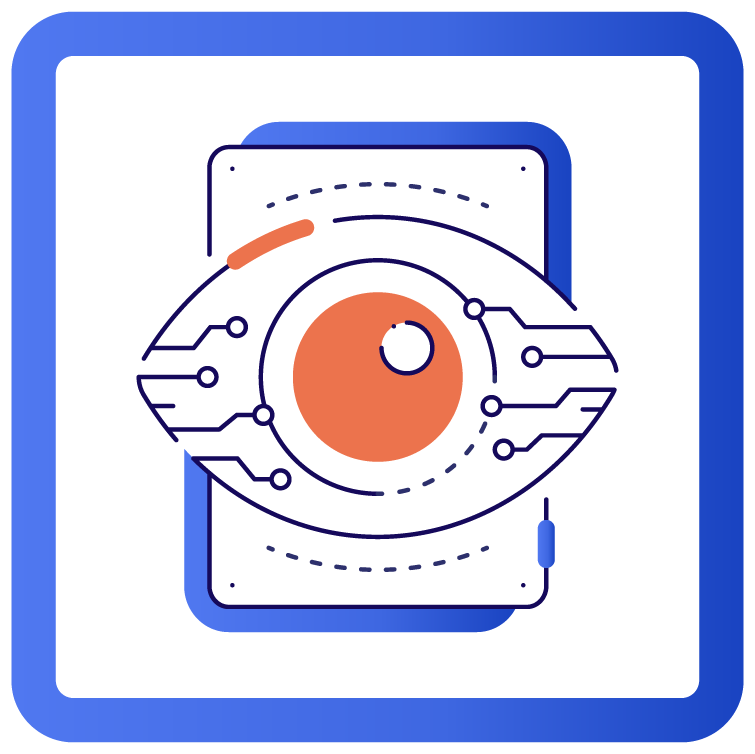
E-commerce businesses often face the challenge of fraud and security threats. Machine learning algorithms act as vigilant guardians by analyzing the transaction data, patterns and user behavior to detect potential fraudulent activities in real-time.
Additionally, machine learning enables customer service automation through chatbots and virtual assistants, providing round-the-clock support and efficient issue resolution.
Key Takeaways:
- Machine learning enables e-commerce platforms to offer personalized experiences to customers.
- Optimizing conversion rates is possible through machine learning-driven data analysis.
- Efficient customer segmentation allows businesses to target customers with personalized marketing strategies.
- Accurate demand forecasting helps businesses manage inventory and optimize pricing effectively.
- Machine learning aids in fraud detection and automates customer service for better efficiency.
Key Use Cases for Machine Learning in E-Commerce
We all know that staying ahead of the competition is the name of the game, and with machine learning, we’ve got the ace up our sleeve. It’s like having an intelligent ally that decodes massive amounts of data, making brilliant moves and insightful decisions, propelling us towards greatness. This powerful innovation is revolutionizing how we sell products, engage customers, and navigate the digital terrain.
From crafting personalized recommendations and finely-tuned marketing strategies to orchestrating seamless inventory management and unearthing fraudulent activities, machine learning is the fuel propelling us to unparalleled success in the e-commerce universe.
In this journey of possibilities, we explore the key use cases where machine learning transforms the ordinary into the extraordinary, creating a remarkable era of efficiency and innovation. So, fasten your seatbelts as we embark on an inspiring voyage to revolutionize e-commerce like never before!
Product Recommendations
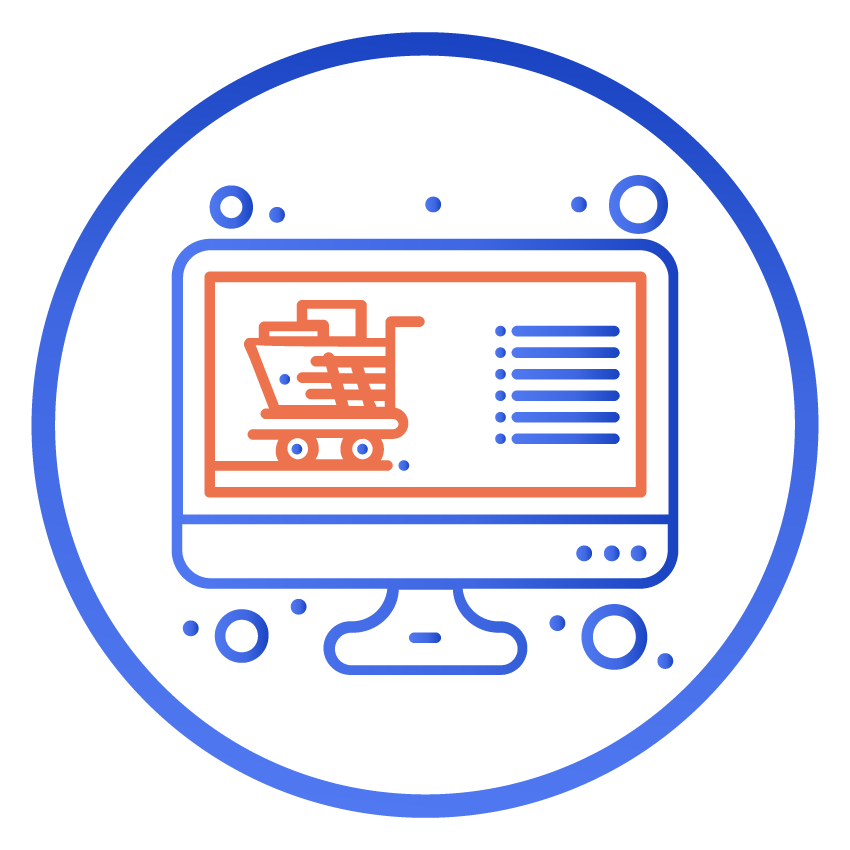
Machine learning revolutionizes product recommendations by understanding each customer’s preferences and behaviors.
By analyzing vast amounts of data, including past purchase history, browsing patterns, and interactions with the website, machine learning algorithms can suggest relevant products to customers.
This personalized approach enhances the online shopping experience, increases the likelihood of making a purchase, and fosters customer loyalty. Whether it’s displaying “Recommended for You” sections or offering complementary products during checkout, product recommendations powered by machine learning create a seamless and engaging online shopping journey.
Demand Forecasting
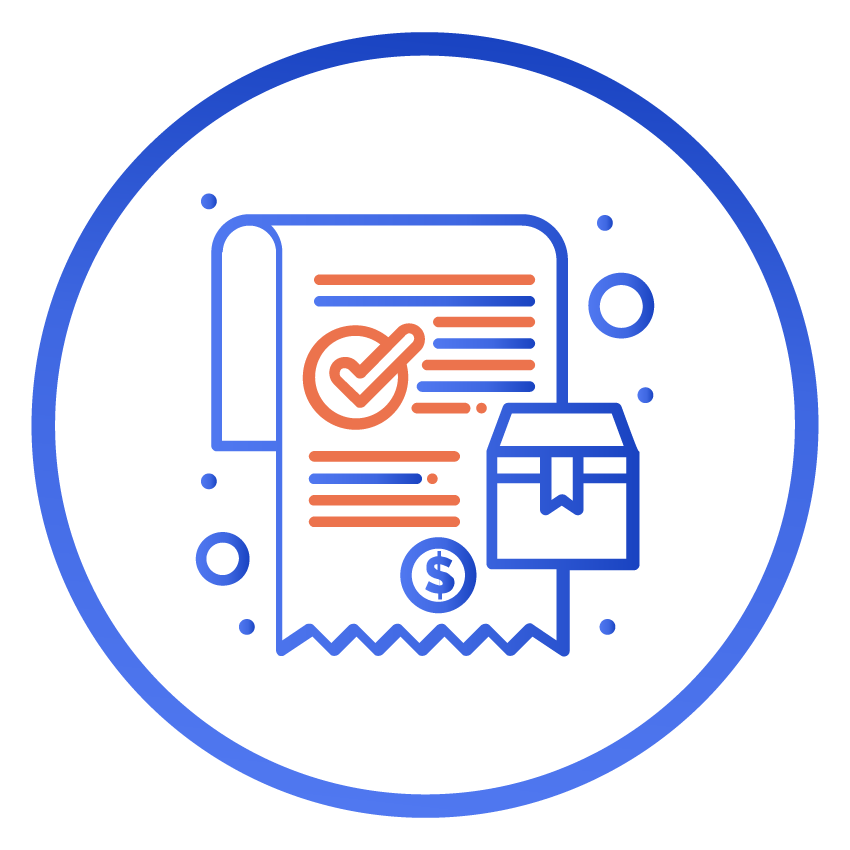
Accurate demand forecasting is critical for e-commerce businesses to optimize inventory levels and meet customer expectations. Machine learning plays a crucial role in this regard by analyzing historical sales data, seasonal patterns, and external factors such as promotions and holidays.
By predicting future demand more accurately, these ecommerce businesses can ensure they have the right products in stock, minimize stockouts, and reduce excess inventory.
This not only saves costs but also improves customer satisfaction by delivering products promptly.
Customer Segmentation
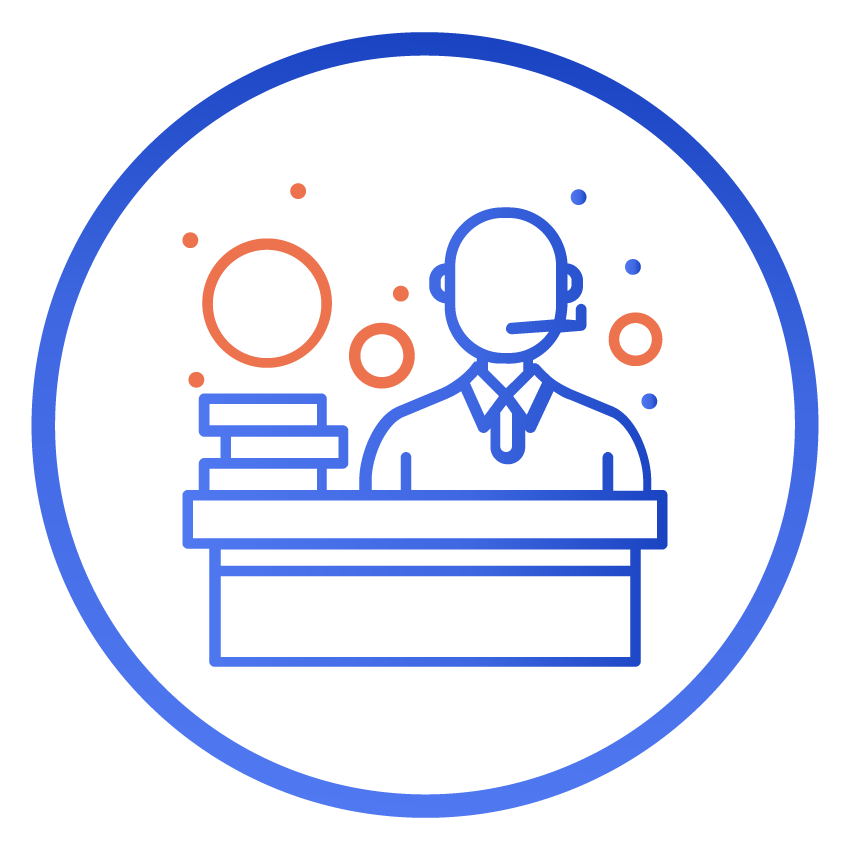
Understanding and segmenting customers based on their behaviors and preferences is vital for targeted marketing and personalized communication.
Machine learning algorithms can group and identify customers with similar characteristics, enabling businesses to tailor their marketing strategies to each segment.
This level of segmentation allows for targeted promotions, personalized content, and relevant offers, leading to higher engagement and conversion rates. Whether it’s segmenting existing customers, based on purchase history or browsing behavior, machine learning empowers businesses to deliver the right message to the right audience.
Fraud Detection
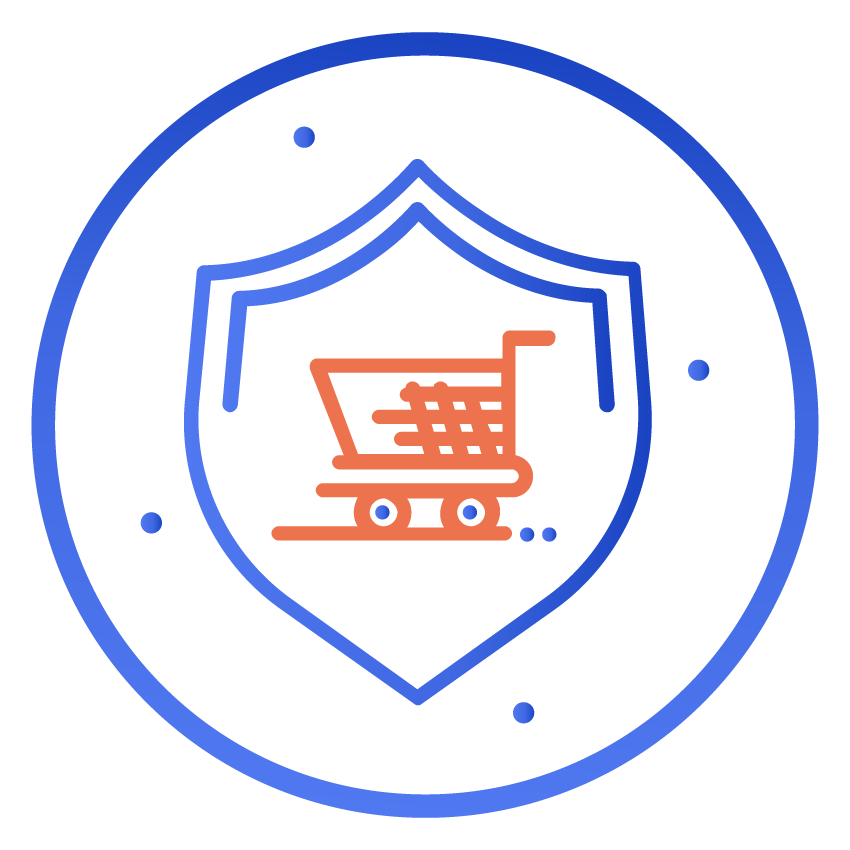
E-commerce platforms are susceptible to fraudulent activities, which can cause significant financial losses and damage customer trust. Machine learning serves as a vigilant guardian by continuously monitoring transactions and user behavior to detect anomalies indicative of fraud.
By analyzing patterns and trends, machine learning algorithms can identify suspicious activities in real-time and trigger security measures to prevent fraudulent transactions.
This proactive approach enhances the an ecommerce platform’s security and protects both businesses and customers from potential threats.
Customer Service Automation
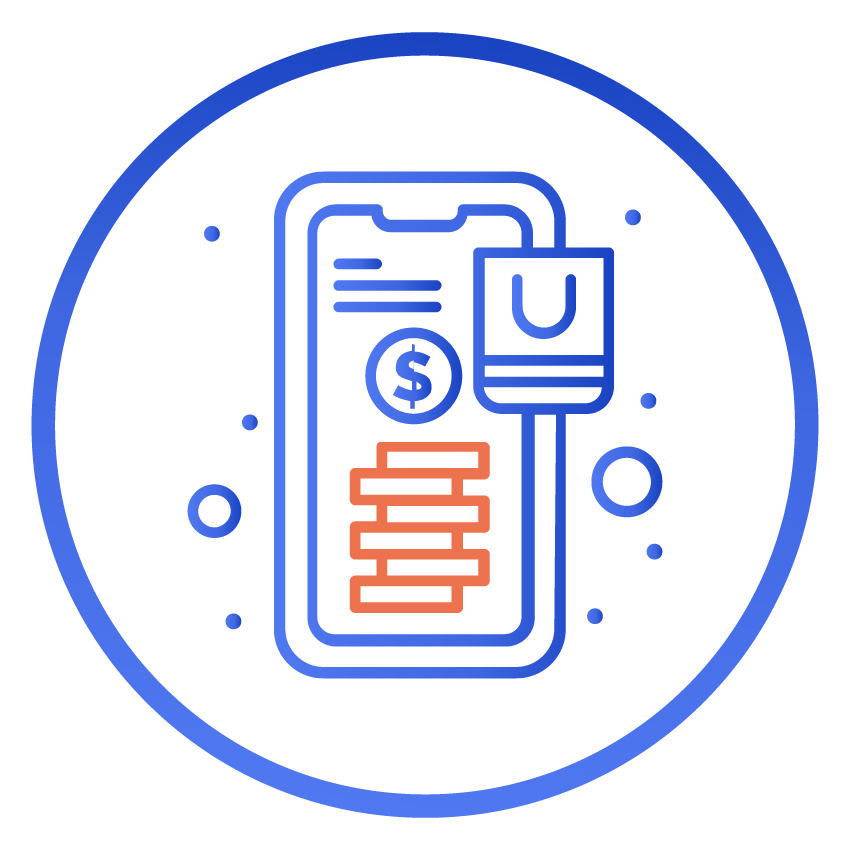
Efficient and responsive customer service is essential for building strong relationships with customers. Machine learning enables businesses to automate customer service through chatbots and virtual assistants.
These AI-driven systems can handle routine queries, provide instant responses, and even assist with order tracking and returns.
By automating these tasks, businesses can significantly reduce response times, improve customer satisfaction, and free up human agents to focus on more complex issues and interactions.
Price Optimization
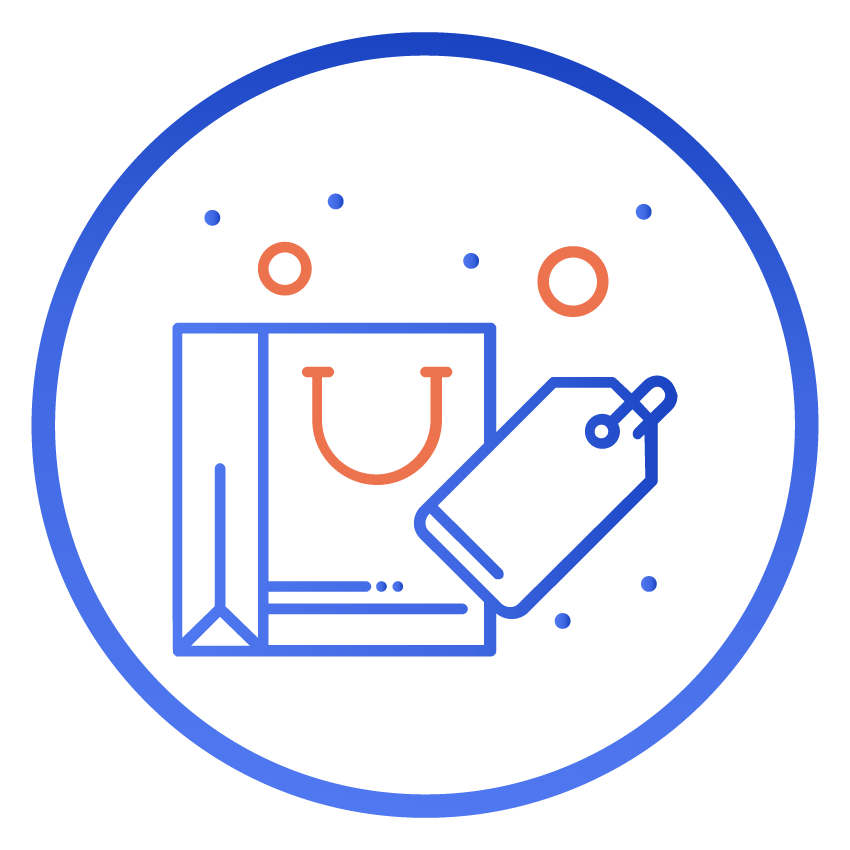
Setting the right prices is crucial for attracting customers while ensuring profitability. Machine learning can optimize pricing strategies by analyzing various factors, including competitor prices, demand elasticity, and customer behavior.
Dynamic pricing algorithms adjust prices in real-time based on these insights, allowing businesses to respond to market fluctuations and increase revenue. This data-driven pricing strategy ensures competitiveness while maintaining profitability in a dynamic market.
Incorporating machine learning in these key use cases empowers e-commerce businesses to deliver personalized experiences, optimize operations, and stay ahead of the competition in a rapidly evolving digital landscape.
Examples of Machine Learning Use Cases in E-Commerce
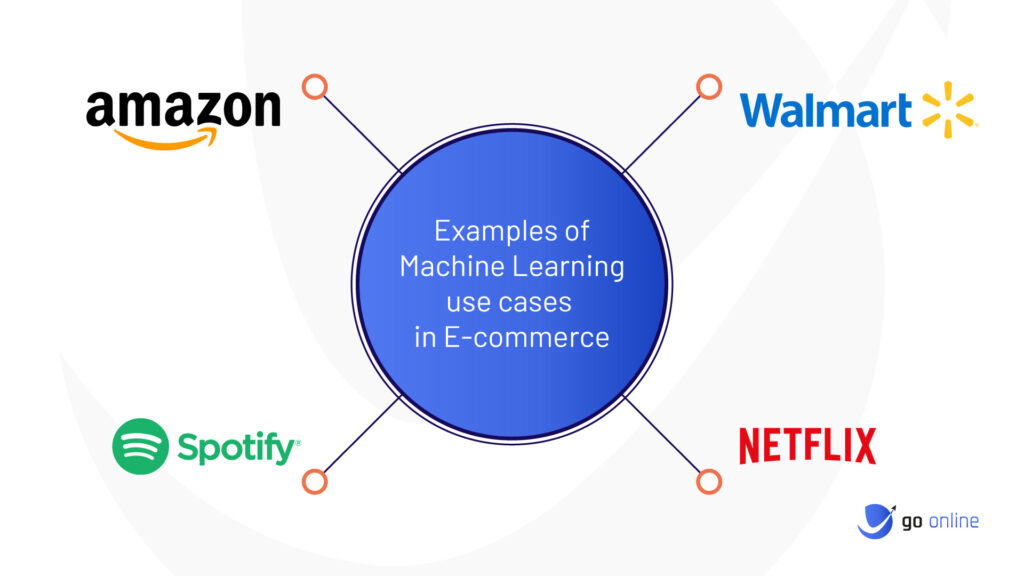
In the ever-evolving landscape of e-commerce, businesses are continuously seeking innovative solutions to elevate their operations, enhance customer experiences, and drive growth. Amidst this pursuit, machine learning emerges as a transformative force, leveraging the potential of data-driven insights and intelligent algorithms to revolutionize the way e-commerce operates.
In this section, we embark on a journey to explore real-world examples of machine learning use cases in e-commerce. These tangible and practical applications illustrate how machine learning empowers businesses to optimize key processes, anticipate customer needs, and craft personalized interactions. By understanding these remarkable use cases, we gain insight into how machine learning shapes the future of online retail, paving the way for unparalleled success.
From enhancing cross-selling opportunities to preventing stockouts, and from optimizing customer segmentation to improving fraud detection, we delve into the specific companies that have harnessed the power of machine learning to create a significant impact in the e-commerce landscape.
Join us as we uncover the innovative strategies employed by leading e-commerce giants to transform their businesses through machine learning. From Amazon’s intelligent product recommendations to Walmart’s proactive inventory management, these examples exemplify the limitless potential of machine learning in revolutionizing e-commerce practices.
Amazon: Enhancing Cross-Selling with Product Recommendations
Amazon, the global e-commerce giant, is renowned for its personalized shopping experience driven by machine learning algorithms. One of their most prominent machine learning use cases is enhancing cross-selling across online stores through product recommendations. When customers browse or purchase a specific product, Amazon strategically displays related or complementary items as suggestions.
For example, when a customer adds a camera to their cart, Amazon’s recommendation engine may showcase relevant accessories like lenses, tripods, or camera bags. By leveraging this smart cross-selling approach, Amazon has seen a substantial increase in average order values and revenue. Customers appreciate the convenience of finding complementary products, while Amazon benefits from increased sales and heightened customer engagement.
Walmart: Preventing Stockouts with Demand Forecasting
Walmart, a global retail giant, utilizes machine learning-based demand forecasting to optimize inventory management and prevent stockouts. With thousands of stores and a vast array of products, efficiently managing inventory is a complex challenge. Walmart’s machine learning algorithms analyze historical sales data, seasonal trends, and external factors like weather patterns and events to accurately predict future demand.
By having a clear understanding of customer preferences and anticipated demand, Walmart ensures that popular products are readily available on their shelves, even during peak seasons or special events. This proactive approach not only maximizes sales opportunities but also enhances customer satisfaction by reducing instances of out-of-stock items.
Spotify: Personalizing Music Recommendations
Although primarily known as a music streaming service, Spotify has a thriving e-commerce component through its personalized music recommendations. Spotify’s machine learning algorithms analyze user listening behavior, genres, and favorite artists to curate personalized playlists and recommend new music.
By using machine learning to understand individual music preferences, Spotify provides a highly personalized and enjoyable listening experience. This not only keeps users engaged with the platform but also encourages them to explore new music and discover emerging artists. As a result, Spotify benefits from increased user retention and a competitive edge in the crowded music streaming industry.
Netflix: Personalizing Movie and TV Show Recommendations
Netflix, the popular video streaming platform, relies heavily on a machine learning algorithm to deliver personalized movie and TV show recommendations to its subscribers. By analyzing user viewing history, movie ratings, social media data, and interactions with the platform, Netflix’s machine learning algorithms create personalized content feeds for each user.
Through this approach, Netflix keeps its vast user base engaged with a curated selection of content tailored to individual preferences. The success of Netflix’s personalized recommendations has been a key driver of its customer retention and global expansion.
Incorporating machine learning in these real-life examples has empowered companies like Amazon, Walmart, Spotify, and Netflix to deliver personalized experiences, optimize operations, less customer churn, and gain a competitive advantage in the ever-evolving world of e-commerce.
What Does This Mean?
In conclusion, machine learning stands as a game-changer in the e-commerce industry, presenting boundless opportunities to elevate businesses to new heights of success. Its transformative impact is evident in the seamless personalized experiences it delivers to customers, resulting in higher conversion rates and increased loyalty.
By harnessing the power of data-driven insights, businesses can optimize their operations, anticipate market demands, and fortify their fraud prevention measures.
The exploration of real-world machine learning use cases in e-commerce showcases the tangible and practical benefits this technology brings to the table. From precision product recommendations to proactive inventory management, machine learning empowers businesses to make informed decisions and stay one step ahead of the competition.
As we delve into these success stories, we gain invaluable insights into how machine learning is reshaping the landscape of online retail, unlocking the potential for unprecedented achievements.
How Amazon Utilized Machine Learning And What Lessons You Can Learn From Them?
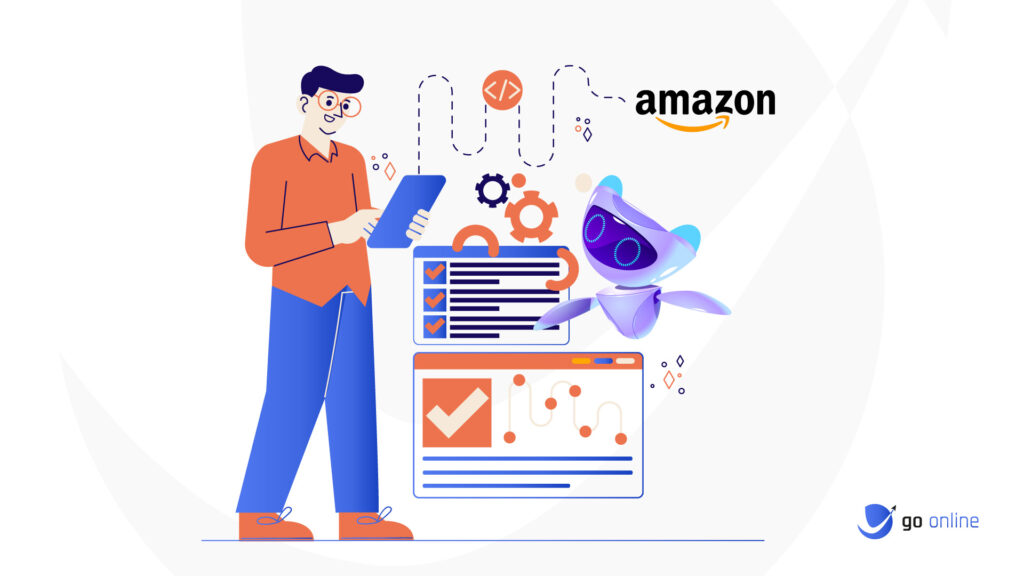
In this section, we delve into a real-life case study showcasing the remarkable impact of machine learning at Amazon, the world’s leading online marketplace.
We will explore how Amazon harnessed the power of machine learning to address key challenges and revolutionize the e-commerce landscape. From personalized product recommendations to demand forecasting and fraud detection, Amazon’s innovative use of machine learning exemplifies the transformative potential of this technology.
Case Study: Amazon – Pioneering Personalization in E-commerce
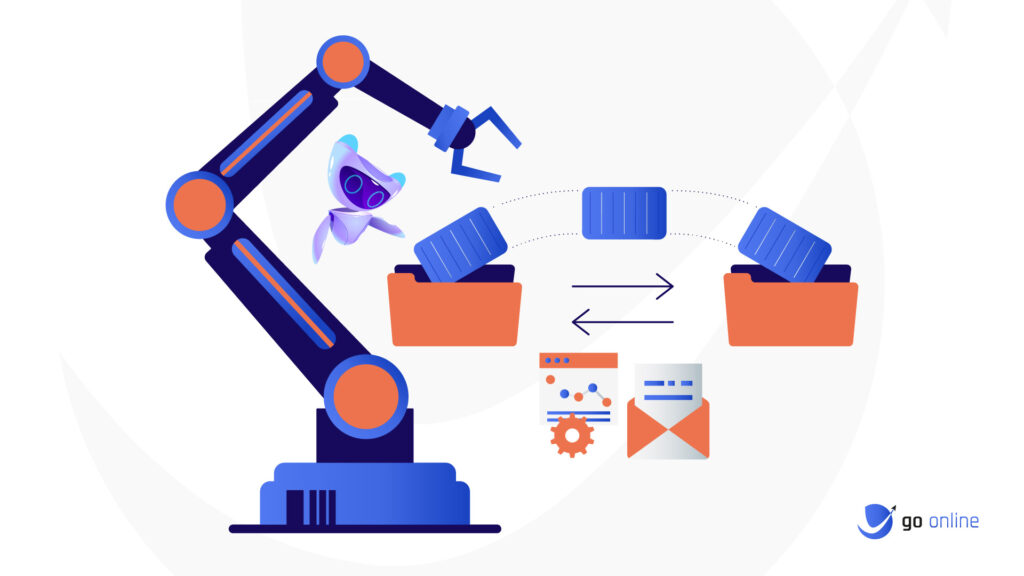
Amazon, the e-commerce giant, faced immense challenges in personalizing the shopping experience for its vast customer base. With millions of products and diverse customer preferences, manually curating tailored product recommendations for each user was an overwhelming task.
Additionally, optimizing inventory management to prevent stockouts and overstocking while meeting customer demand required precise and data-driven strategies.
To address these challenges and maintain their position as an industry leader, Amazon recognized the need to embrace machine learning-driven solutions.
Machine Learning Implementation and Effects Discussing the Motivation for Implementing Machine Learning and Setting Business Goals
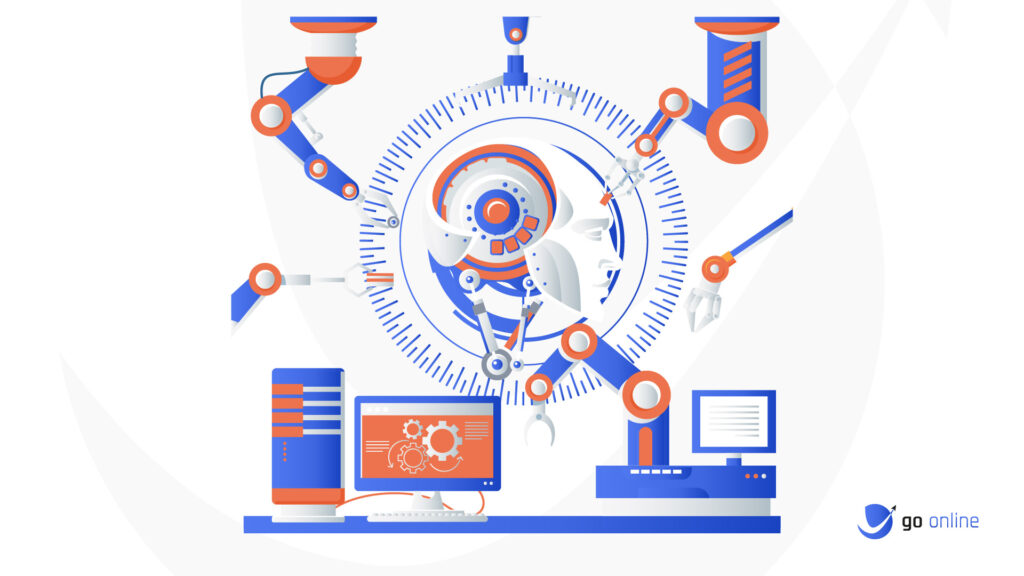
Motivated to elevate customer experiences, improve operational efficiency, and drive revenue growth, Amazon embarked on an ambitious journey to implement machine learning across their e-commerce operations.
The business goals were set as follows:
- Enhanced Personalization: Leverage machine learning algorithms to analyze customer behavior, purchase history, and browsing patterns to deliver highly personalized product recommendations.
- Efficient Demand Forecasting: Utilize machine learning insights to accurately forecast customer demand, optimizing inventory management and minimizing stockouts.
- Robust Fraud Detection: Implement machine learning algorithms to proactively detect and prevent fraudulent activities, safeguarding both Amazon and its customers.
Description of the Machine Learning Implementation Process
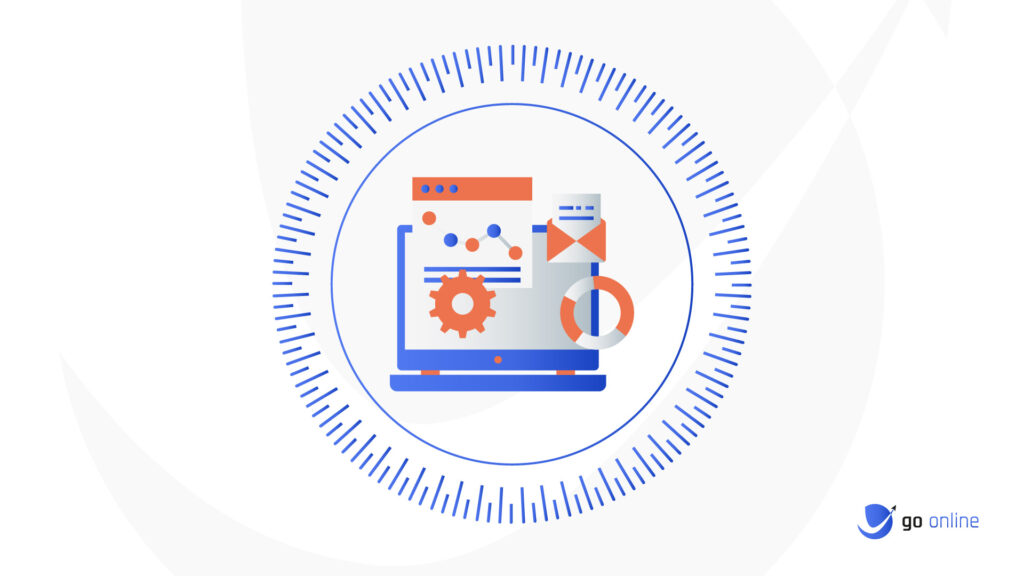
Amazon initiated the machine learning implementation by consolidating and processing vast amounts of customer data from diverse sources. Leveraging cloud-based infrastructure, they streamlined data storage and processing for real-time insights.
For personalized product recommendations, Amazon employed collaborative filtering and deep learning models to understand user preferences and suggest relevant products. Demand forecasting involved time series analysis and regression models to predict future demand based on historical data and external factors.
To address fraud detection, Amazon utilized anomaly detection algorithms and machine learning-based pattern recognition to identify suspicious activities and take preventive measures.
Presentation of the Effects of the Implementation
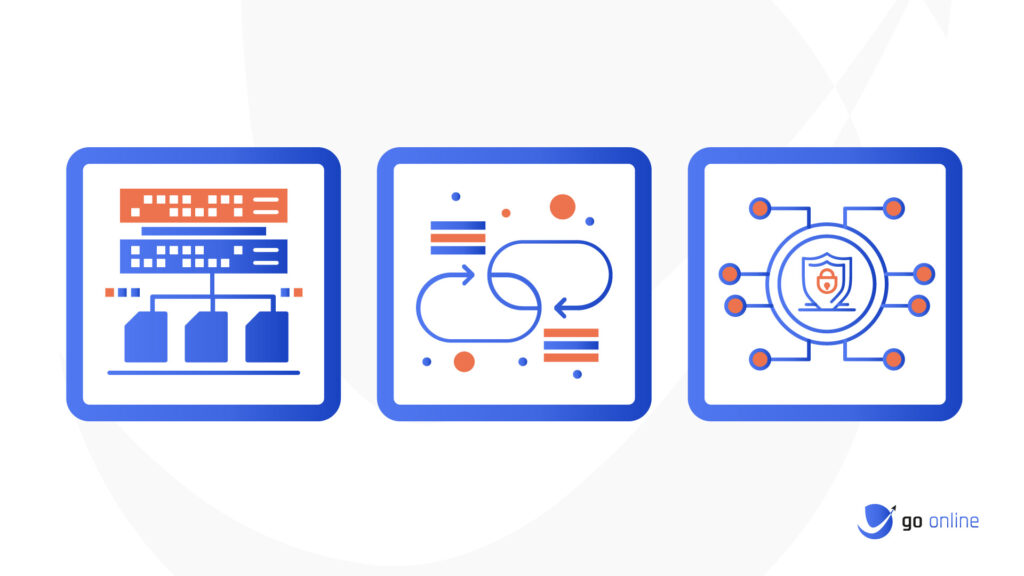
The implementation of machine learning had a profound and transformative impact on Amazon’s e-commerce operations, revolutionizing the way the company interacted with its customers and managed its business processes. Here are some key aspects where the effects were particularly remarkable:
1. Increased Personalization:
With machine learning-powered product recommendations, Amazon witnessed a substantial increase in customer engagement and satisfaction. Customers enjoyed a tailored shopping experience, resulting in higher conversion rates and increased customer loyalty.
2. Efficient Demand Forecasting:
By using data science for accurately predicting customer demand, Amazon significantly improved inventory management. They minimized stockouts and overstocking, leading to reduced costs and improved customer satisfaction.
3. Robust Fraud Detection:
Machine learning-driven fraud detection systems helped Amazon proactively identify and prevent fraudulent activities. This proactive approach protected both the company and its customers, enhancing trust and security.
To wrap up, Amazon’s case study showcases the immense potential of machine learning in driving significant improvements across e-commerce operations. By strategically implementing a machine learning solution, businesses can enhance personalization, optimize demand forecasting, and fortify security, ultimately positioning themselves as leaders in the competitive e-commerce landscape. Embracing machine learning is not just a technological advancement; it’s a transformative tool that empowers all ecommerce companies to thrive and deliver exceptional shopping experiences to their customers.
Conclusion
Machine learning technology has revolutionized the e-commerce industry, offering numerous opportunities to enhance customer experiences and drive business success. By analyzing vast amounts of data, machine learning algorithms enable personalized shopping recommendations, optimized search engines, and efficient fraud detection, fostering trust and loyalty among customers.
This technology also facilitates demand forecasting, enabling businesses to manage inventory effectively and prevent stockouts during peak periods. As a result, machine learning has become a cornerstone for e-commerce growth and competitiveness.
Furthermore, machine learning’s impact on ecommerce sales extends beyond customer-facing aspects, permeating backend operations and supply chain management. With the ability to analyze complex data sets, machine learning optimizes logistics and inventory management, ensuring seamless order fulfillment and minimizing delivery times.
Additionally, it aids in dynamic pricing strategies, allowing e-commerce businesses to adapt to market fluctuations and competitor pricing in real-time, maximizing profitability and competitiveness. Embracing machine learning has become imperative for e-commerce businesses seeking to thrive in a data-driven and hyper-competitive landscape, as it empowers them to stay agile, customer-centric, and at the forefront of innovation.
As this technology continues to evolve, its potential for reshaping the e-commerce industry remains limitless.
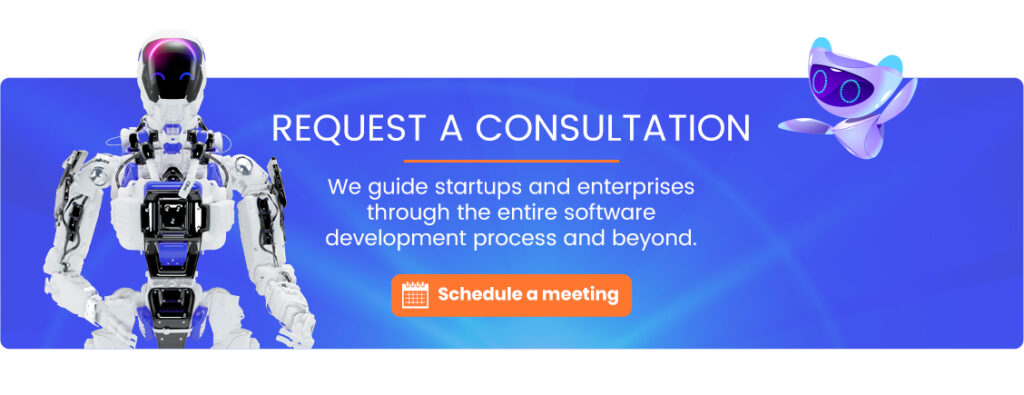