As manufacturing companies strive to stay ahead of the competition, they’re turning to AI for an extra edge. One tool, in particular, is gaining traction – AI based visual inspection, or so-called AI defect detection.
With the power to detect defects that might be missed by other methods, this technology is quickly becoming a must-have for any manufacturing company looking to optimize their production processes, reduce costs, and improve product artificial intelligence in quality control.
The possibilities for using machine learning defect detection are almost limitless, as it can be tailored to the specific needs and requirements of each industry and application.
What is AI Defect Detection?
AI defect detection is an innovative technology for identifying defects in products during the production process. It uses computer vision to analyze product images to further enhance defect detection and find anomalies in shape, dimensions, color, and texture.
Using machine learning algorithms, AI deep learning inspection can analyze historical and real-time data to detect subtle differences in product characteristics that may indicate a defect.
This manufacturing defect analysis technology is vital for modern manufacturing companies as it can dramatically reduce waste and quality control costs while simultaneously increasing production speed and accuracy. Most importantly, this system is great for reducing costs when it comes to defect detection using computer vision.
What AI Defect Detection Is Capable Of?
Some examples of defects that AI defect detection can detect include:
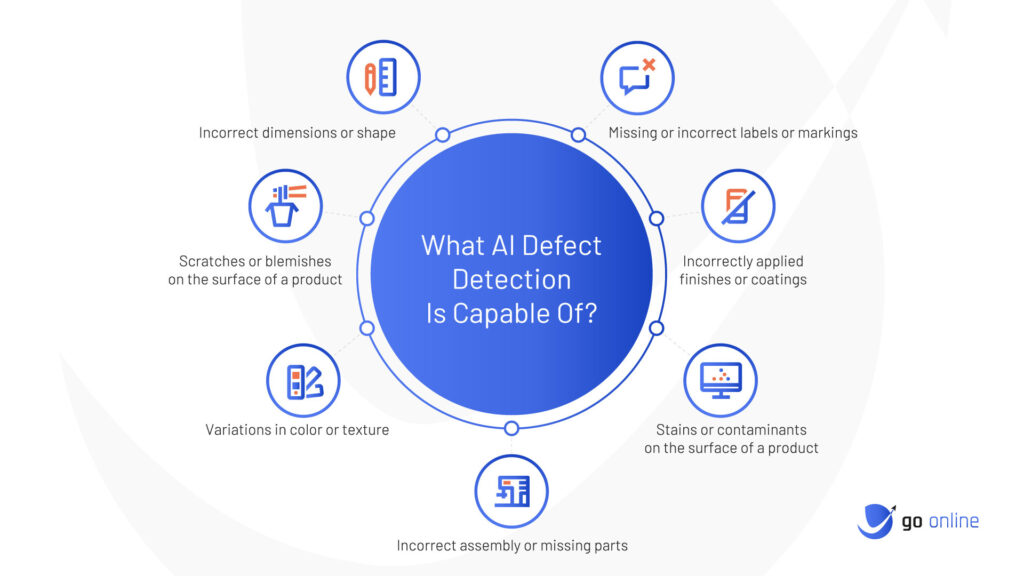
Overall, this type of automated defect detection can be used to detect a wide range of defects. The specific defects that can be detected will depend on the capabilities of the AI-based defect detection system and the characteristics of the products being produced.
With the power of AI and machine learning, the potential for AI defect detection to identify and prevent defects is virtually limitless.
Applications Where AI Defect Detection Delivers the Best Results
AI defect detection can be more profitable. Some examples include:
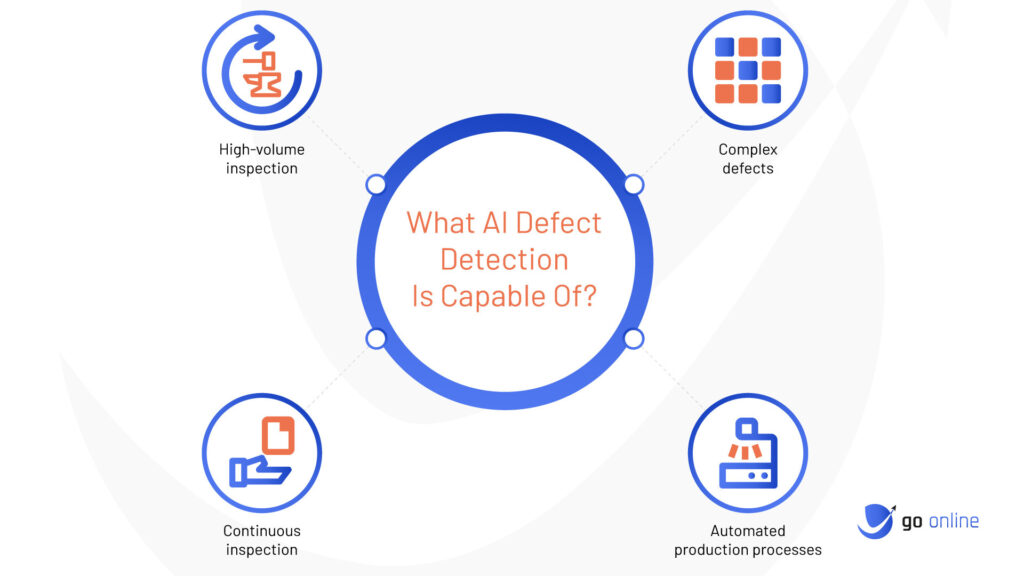
- High-volume inspection: If a company has a high volume of products to inspect, it can be more efficient and cost-effective to use an AI system to quickly and accurately analyze the data.
- Complex defects: AI systems can be trained to recognize complex patterns that might be difficult for humans to detect.
- Continuous inspection: AI systems can operate continuously, potentially identifying defects in real time.
- Automated production processes: In automated production processes where products are continuously moving through the production line, it can be more efficient to use an AI system to increase defect detection using machine learning.
Types of AI Defect Detection
There are several types of AI-based defect detection systems, each with its strengths and limitations. Some examples include:
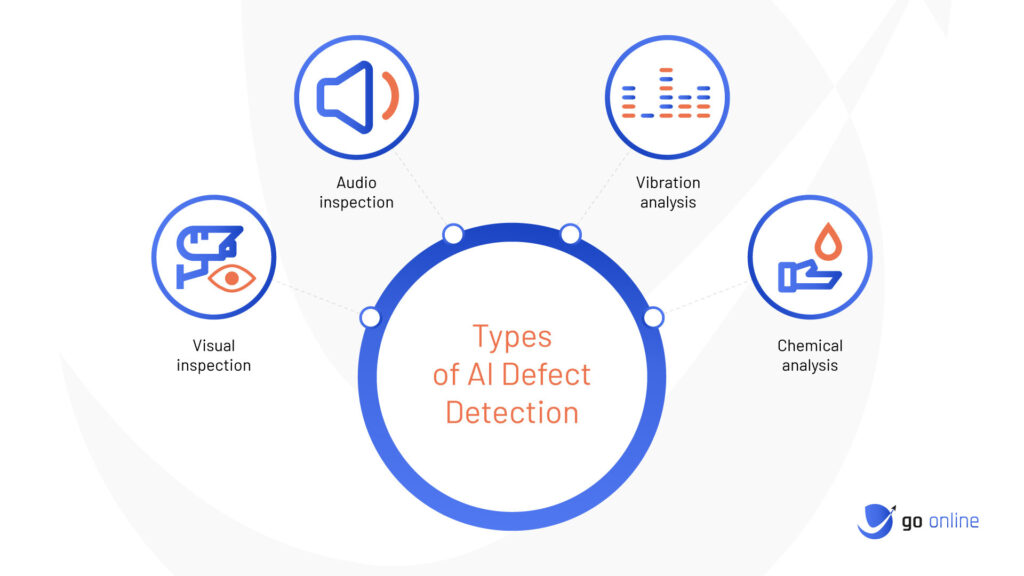
Visual inspection
Automated visual inspection AI systems use image processing algorithms to analyze images or video feeds to increase defect detection. This can be particularly effective for identifying defects in physical products or structures.
Audio inspection
AI-based audio inspection systems use machine learning quality control algorithms to analyze audio signals to identify defects. This can be particularly effective for identifying defects in machinery or other equipment that produce distinctive sounds when operating correctly or incorrectly.
Vibration analysis
AI-based vibration analysis systems use machine learning algorithms to analyze vibrations in machinery or other equipment to identify defects. This can be particularly effective for identifying defects in machinery or other equipment that produce distinctive vibration patterns when operating correctly or incorrectly.
Chemical analysis
AI-based chemical analysis systems use visual inspection machine learning algorithms to analyze chemical compositions to identify defects. This can be particularly effective for identifying defects in chemical processes or products, such as pharmaceuticals.
Which Industries Can Benefit From AI Defect Detection?
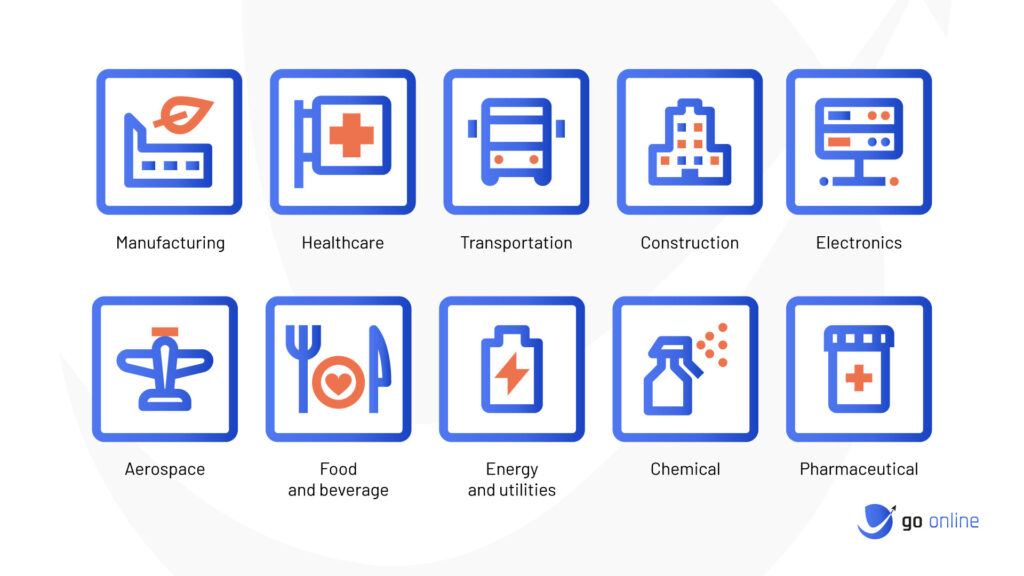
The possibilities are virtually endless, as AI defect detection can be applied to a wide range of products to defect detection in manufacturing.
In this section, we will take a closer look at some of the industries that can benefit from quality control camera systems detection and the specific ways in which this technology can be used to improve their operations.
Let’s have a look at a few examples:
Manufacturing
In the manufacturing industry, defects in products or production process can lead to costly rework, scrapped parts, and customer dissatisfaction. AI defect detection systems can help to identify and fix anomaly detection in manufacturing process early in the production process, improving efficiency and reducing the overall cost of defects.
This is valuable for industries with high-volume inspection or complex defects, such as automotive manufacturing, electronics manufacturing, and aerospace manufacturing. In that light, AI damage detection comes in quite handy.
Healthcare
In the healthcare industry, defects in medical devices or pharmaceuticals can have serious consequences. AI defect detection systems can help to identify and fix early in the production process, reducing the risk, and harm and improving the overall safety of medical products.
This is particularly valuable for industries with complex defects or strict regulatory requirements. These include medical device manufacturing and pharmaceutical manufacturing, and quality control vision systems are able to do deep learning inspection.
Construction
Defects in buildings or infrastructure can lead to costly repairs, accidents, and customer dissatisfaction. AI quality control machine learning can help to identify and fix defects early in the construction process, improving efficiency and reducing the overall cost of defects.
This is valuable for industries with complex defects or strict regulatory requirements, such as building construction and civil engineering.
How to Implement a AI Visual Inspection System Into Your Business?
Integrating a machine vision quality control system using artificial intelligence involves several steps. Here is a detailed description of the process:
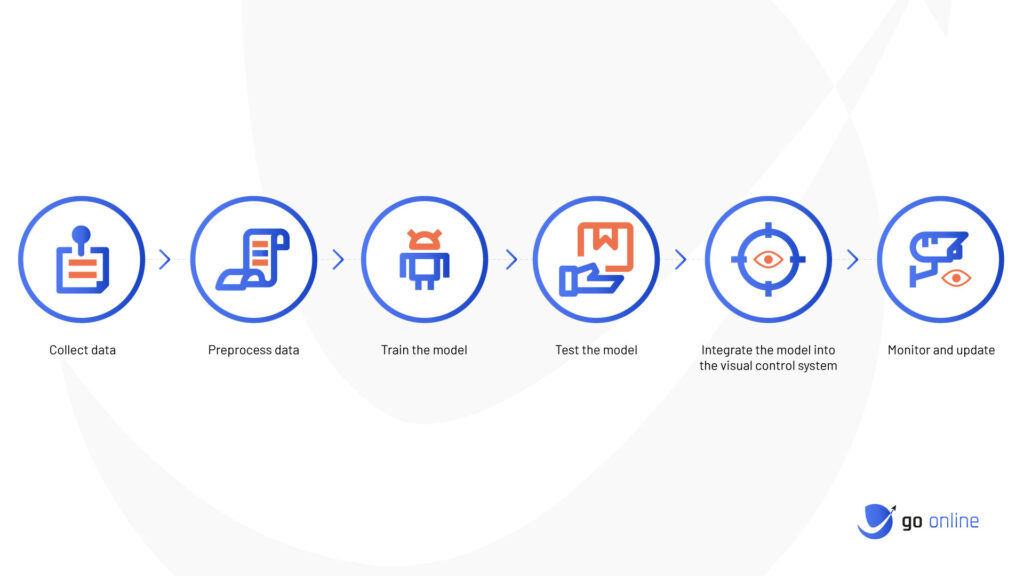
- Collect data: The first step in integrating a visual control system is to collect data on what defects look like. This may involve taking photographs or videos of the defects, or it may involve collecting other types of data, such as measurements or sensor readings.
- Preprocess data: The collected data may need to be cleaned and formatted before it can be used to train the AI model. This may involve removing any irrelevant data, filling in missing values, or scaling the data to a consistent range.
- Train the model: Once the data is prepared, it can be used to train an AI to develop a deep learning model to identify critical defects. This typically involves feeding the data into the model and adjusting the model’s parameters to minimize the error between the model’s predictions and the actual defects in the data.
- Test the model: After the learning model has been trained, it can be tested on a separate set of data to see how well it performs on previously unseen examples. This helps to ensure that the model is not overfitting to the training data and can generalize to new situations.
- Integrate the model into the visual control system: If the deep learning model development performs well on the test data, it can be integrated into the visual control system. This may involve installing the model on a computer or device that is connected to the visual control system, or it may involve integrating the model into the system’s computer vision quality inspection or hardware, for defect detection using computer vision.
- Monitor and update: The deep learning model functionality should be monitored over time to ensure it is still performing well and to identify any issues that may arise. The model may also need to be updated periodically with new data, so the AI based visual inspection can ensure it performs well.
Here Is an Example of How This Process Might Work in Practice:
Imagine that you are integrating a visual control system to inspect automotive parts for defects. The first step would be to collect data on what defects look like. This might involve taking photographs of scratched, dented, or otherwise imperfect parts. You would then preprocess this data to ensure it is clean and properly formatted for training the AI model.
Next, you would train the AI model on this data, adjusting its parameters to minimize the error between its predictions and the actual defects in the data, especially when it comes to machine learning quality control.
After the model has been trained, you would test it on a separate set of data to ensure that it is not overfitting to the training data and can generalize to new situations.
If the model performs well on the test data, you would integrate it into the visual control system, which might involve installing it on a computer connected to a camera that is used to inspect the parts.
Finally, you would monitor and update the model as needed to ensure it continues to perform well.
What Are the Advantages of AI Defect Detection?
AI-based defect detection systems are quickly becoming an essential tool for manufacturers looking to improve the efficiency and quality of their production processes. Here are some benefits of using an AI-based defect detection system:
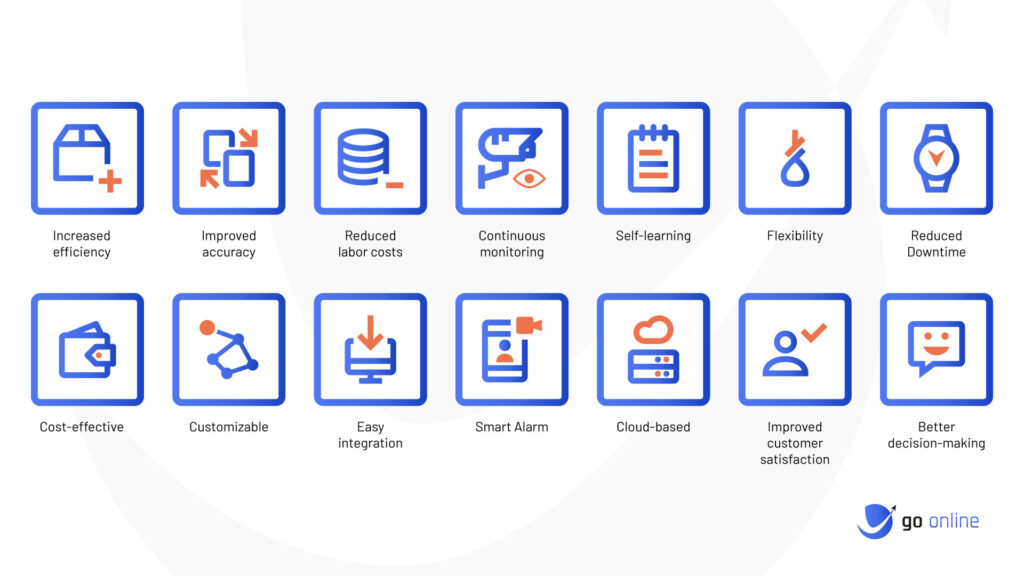
- Increased efficiency: With the implementation of AI-based defect detection, you will be able to increase manufacturing defect analysis of large amounts of data quickly and accurately, allowing for faster defect detection in manufacturing.
- Improved accuracy: By using an AI-based system, you will see an improvement in accuracy as the system learns from its past experiences and reduces the number of false positives and false negatives.
- Reduced labor costs: Automating the defect detection process with an AI-based system will help you to reduce the need for manual inspection, resulting in cost savings for your labor and resources. An AI-based system can be scaled up or down to meet your specific needs, making it more cost-effective than other types of inspection systems.
- Continuous monitoring: An AI-based system can be designed to continuously monitor your production lines’ defect detection computer vision, providing real-time detection of defects and allowing for immediate corrective action.
- Self-learning: The AI-based system will learn from the feedback provided and improve its performance over time, allowing you to see continuous improvement in the system because of deep learning manufacturing.
- Flexibility: An AI-based system can be integrated into a variety of your manufacturing processes, from printed circuit board assembly to injection molding, giving you a wide range of coverage in your production line.
- Reduced Downtime: By using an AI-based system, you will be able to detect defects quickly and accurately, reducing downtime for repairs and maintenance, thus increasing overall productivity for your manufacturing process.
- Cost-effective: AI-based defect manufacturing defect detection systems can be more cost-effective than traditional inspection methods, especially when used in high-volume manufacturing environments.
- Customizable: AI-based systems can be tailored to meet the specific needs of a particular manufacturing process, allowing for greater flexibility in terms of the types of defects that can be detected.
- Easy integration: AI-based systems can be integrated into existing manufacturing processes and equipment, which makes implementation and adoption faster and more efficient.
- Smart Alarm: With the integration of AI-based systems, you can set smart alarms and notifications, which can be alerted in real time when an abnormal event occurs.
- Cloud-based: AI-based systems can be cloud-based, which allows for easy access and data storage solution, in addition, they can support remote access to monitoring and controlling the process from a different location.
- Improved customer satisfaction: By detecting defects early in the production process, manufacturers can ensure that only high-quality products reach the customer, which can help to improve customer satisfaction.
- Better decision-making: By using AI-based systems, manufacturers can analyze large amounts of data and make better-informed decisions about how to improve their production processes and reduce the incidence of defects.
In conclusion, AI-based defect detection systems can offer many advantages to manufacturers, such as increased efficiency, improved accuracy, and cost savings. Furthermore, quality control machine helps to improve the quality of products and services, enhance customer satisfaction, and trace the process for future reference. So, if you’re wondering how to reduce defects in manufacturing, this is the right way to go.
AI Defect Detection Challenges and Limitations
There are some challenges and limitations that manufacturers should be aware of when implementing such a system. So, let’s take a look at what is an advantage of the anomaly detection method.
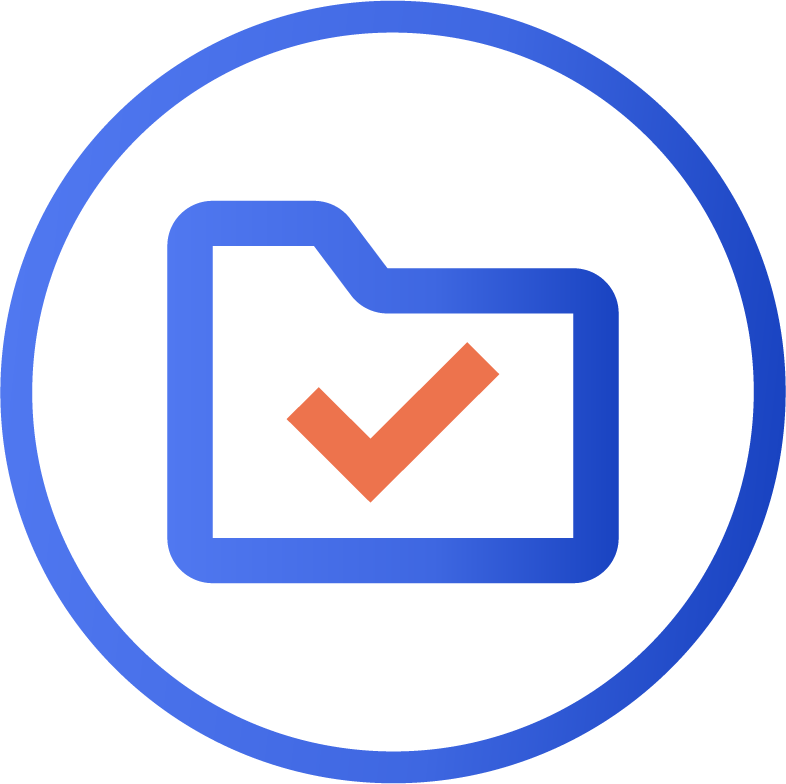
Data quality: One of the main challenges of AI-based defect detection is obtaining high-quality training data. If the data is incomplete, inconsistent, or biased, the automated visual inspection system in manufacturing industries may not perform as well as expected.
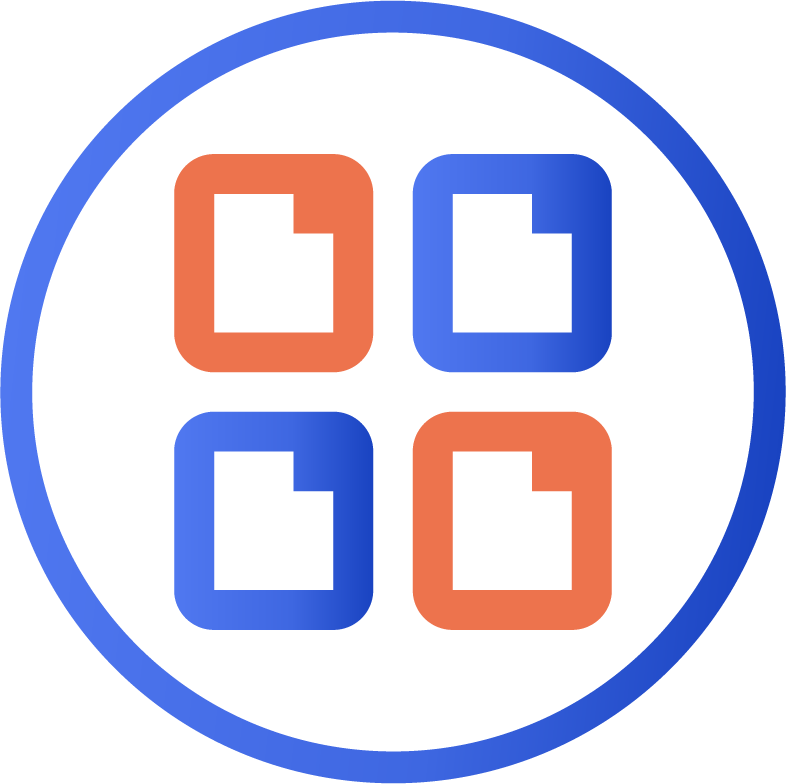
Initial Investment: Implementing AI-based systems can be costly. It is not only the cost of the hardware, software, and installation, but also the cost of data labeling, data management, and maintenance of the system.
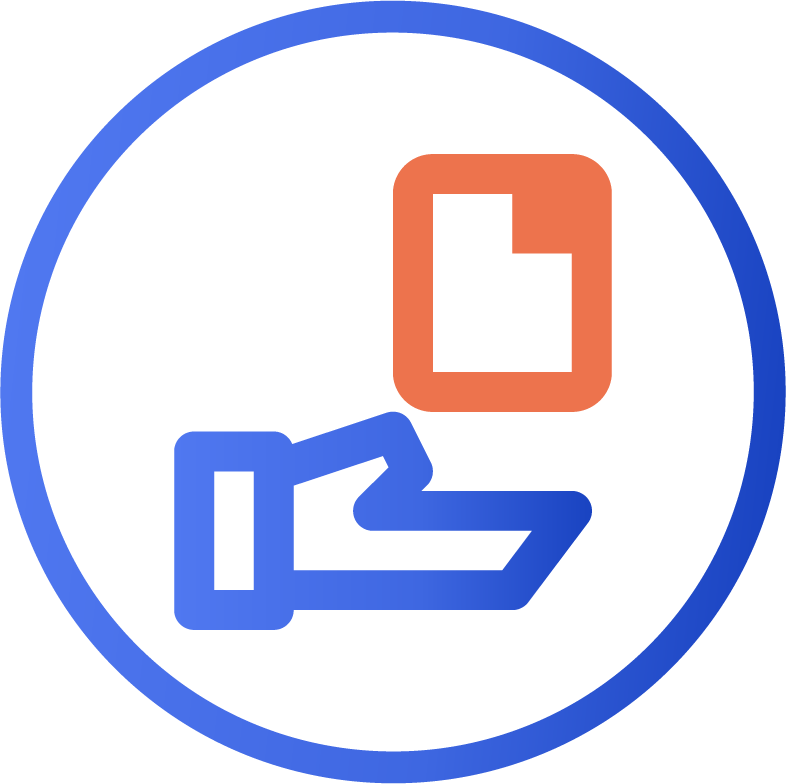
Explaining the decision-making process: AI-based systems can be difficult to understand and interpret the reasoning behind their decisions, which can make it hard to debug and troubleshoot the system.
It’s important to keep in mind that these challenges may vary depending on the specific application and the state of technology at a specific time.
How Does AI Defect Detection Work?
AI defect detection is a process in which AI quality control camera systems are used to identify defects or abnormalities in products, construction projects, medical images, or other applications. These defects can be physical imperfections, such as scratches or dents, or they can be deviations from specifications or blueprints.
To use AI for defect detection, a dataset of examples showing what defects look like is collected. This data may come from manual inspections of products or other sources, such as medical images or construction plans. The data is then preprocessed to ensure that it is clean and properly formatted for use in training the AI model.
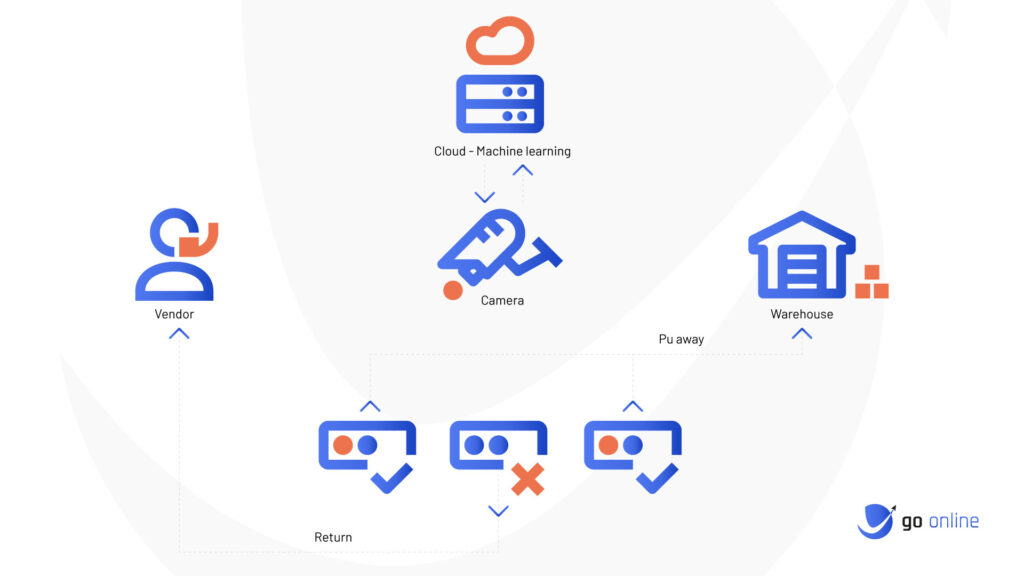
The AI model is then trained on this data to learn what defects look like. This is typically done by feeding the data into the model and adjusting the model’s parameters to minimize the error between the model’s predictions and the actual defects in the data. The model is then tested on a separate set of data to ensure that it is not overfitting the training data and can generalize to new situations.
If the model performs well on the test data, it can be deployed in the real world to identify defects in the application of interest. The model’s performance should be monitored over time to ensure it is still performing well and to identify any issues that may arise. The model may also need to be updated periodically with new data to ensure it continues to perform well.
Let’s Take a Look at an Example:
One manufacturing defect example of the application is the automatic recognition of defects on the surface of solar panels. The neural network could be used to analyze photos of solar panels to identify different types of defects such as cracks, scratches, debris, etc.
To train such a neural network processing, a large training test dataset is needed, which includes pictures of solar panels with and without defects of various types. The network learns to recognize different deep learning models based on this data. Then, it can be used to analyze new photos of solar panels and detect defects in them with the help of manufacturing computer vision.
Other Examples of AI Defect Detection Applications
Here are some examples of how you can use machine vision in manufacturing to identify defects:
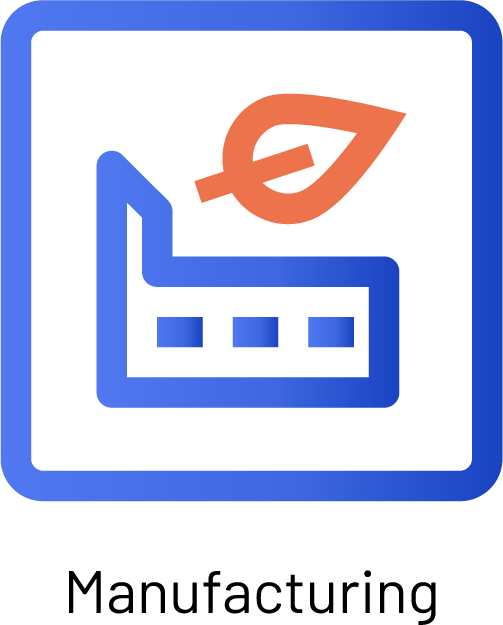
In manufacturing, it can be used to identify product defects during the manufacturing process. For example, anomaly detection in manufacturing can be used to recognize scratches, dents, or other imperfections in car parts during manufacture. Simply said, computer vision for manufacturing is great for anomaly defects.
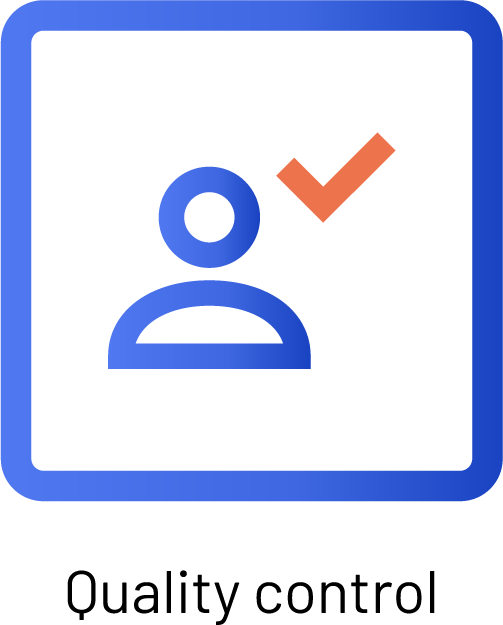
Artificial intelligence in quality control can be used to check products for defects before they are shipped to customers. For example, it can be trained to use computer vision for quality control to identify abnormalities, such as smartphones with cracked screens.
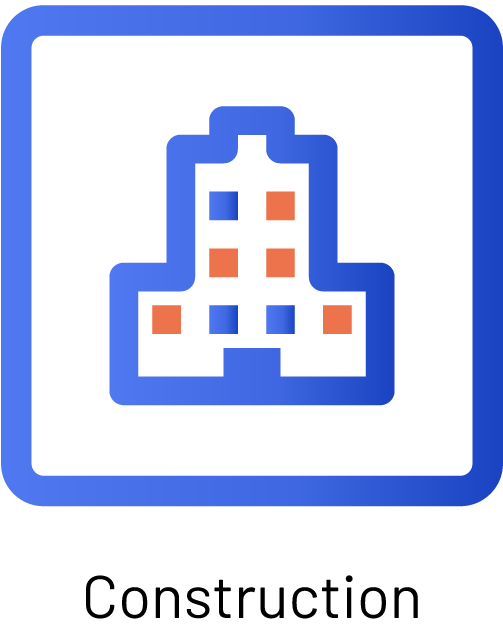
Can be used to identify defects in construction projects, such as cracks in concrete or deviations from plans.
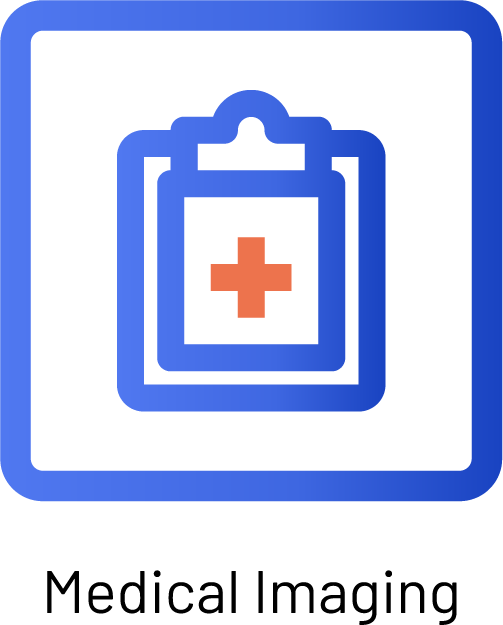
And it can be used to detect abnormalities in medical images such as X-rays or MRIs. For example, they may be trained to recognize signs of cancer or other diseases in medical images.
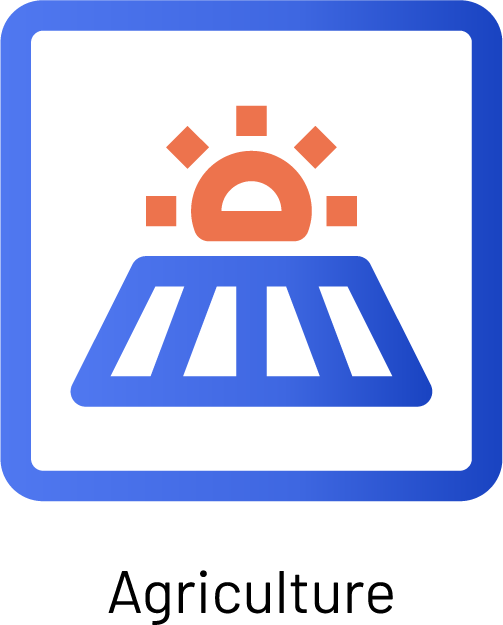
It can be used to identify defects in crops, such as diseased plants or pests, and to optimize irrigation and fertilization to improve crop yields.
In each of these examples, my task is to analyze the data and identify defects that can be difficult or time-consuming to detect by a human. Automating the AI damage detection process can help improve automated inspection efficiency and accuracy and free up data science engineers to focus on more complex tasks.
Methods That Can Be Used to Detect Defects Based on Artificial Intelligence
Here are several methods that can be used to detect defects using artificial intelligence:
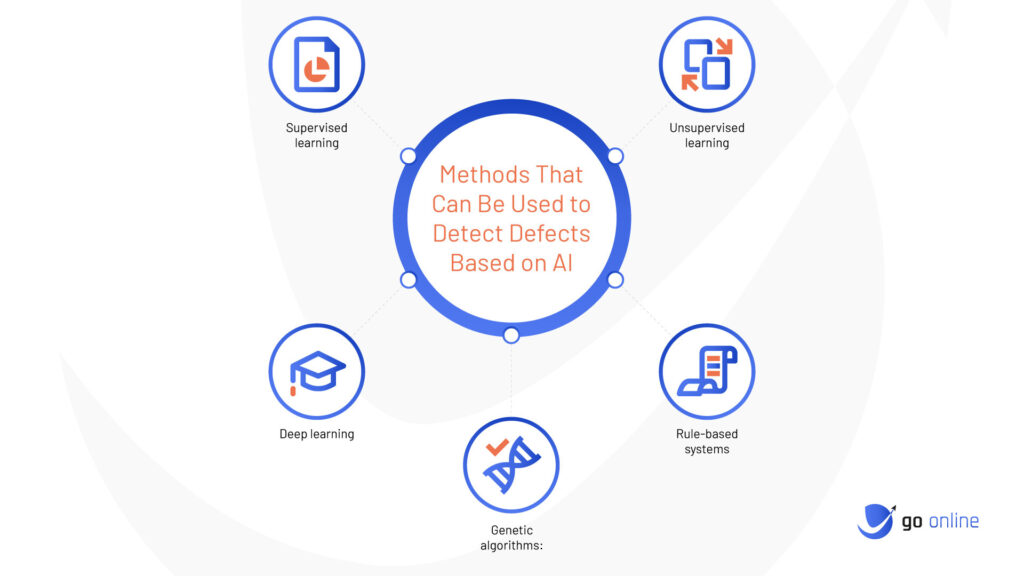
- Supervised learning: In supervised learning, the AI model is trained on a labeled dataset of examples that show what defects look like. The AI visual inspection model is then tested on a separate set of data to ensure that it can be generalized to new situations.
- Unsupervised learning: In unsupervised learning, the AI visual inspection system model is not given any labeled examples of defects. Instead, it is trained to identify patterns and anomalies in the data. This can be useful for detecting defects that are not easily identifiable by humans.
- Deep learning: Deep learning in manufacturing is a type of machine learning model that uses neural networks to learn complex patterns in data. With automated defect detection using machine learning, identifying defects in images, such as medical images or construction plans, by using the defect detection image processing method is guaranteed.
- Rule-based systems: Rule-based systems use a set of predetermined rules to identify defects. For example, a rule-based system might be programmed to identify cracks in concrete that are wider than a certain threshold.
- Genetic algorithms: Genetic deep learning algorithms are a type of optimization algorithm that mimics the process of natural selection to find solutions to problems. They can be used to identify defects by searching for the optimal combination of parameters that minimize the error between the model’s predictions and the actual defects in the data.
These are just a few examples of methods that can be used for defect detection using artificial intelligence.
Conclusions
In conclusion, AI-based defect detection systems can offer many advantages to manufacturers looking to improve the efficiency and quality of their production processes. These quality control vision systems can analyze large amounts of data quickly and accurately, allowing for faster detection of defects, reducing the need for manual inspection, and decreasing the number of false positives and negatives.
They can also be trained to detect a wide range of different types of defects, making them adaptable to a variety of manufacturing processes. Additionally, AI-based systems can be designed to continuously monitor production lines, providing real-time detection of defects, and allowing for immediate corrective action.
Despite the challenges and limitations, investing in an AI-based defect detection system can be a strategic decision for manufacturers looking to improve their overall production process and increase the quality of their products.
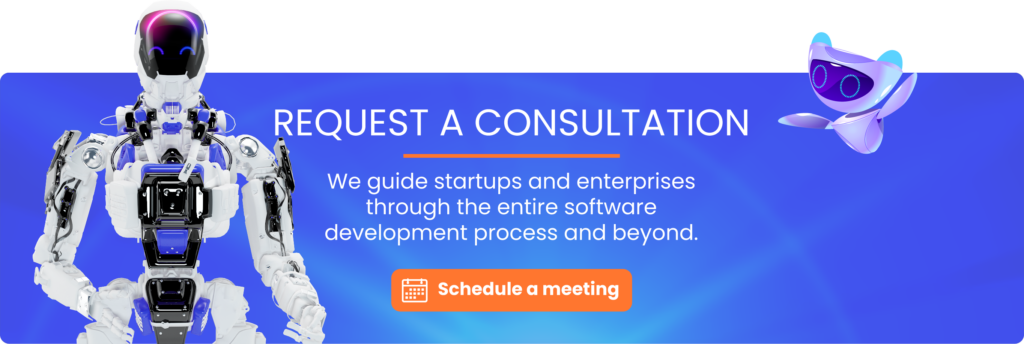